The Ripple Effect Of Poor Data Quality
-
- Lead Generation and Qualification: Inaccurate or incomplete lead data can lead to wasted sales efforts, targeting the wrong audience, and missed opportunities. For example, a telecommunications company might spend significant resources on marketing campaigns targeting customers with incorrect contact information, leading to low conversion rates and decreased ROI.
- Quoting and Proposal: Errors in product information, pricing, or customer details can result in incorrect quotes, delayed proposals, and lost deals. In the software industry, inaccurate pricing information can lead to lost deals due to competitors offering better pricing based on correct data.
- Order Processing: Incorrect customer or shipping information can cause order delays, returns, and customer dissatisfaction. A retail company with poor address data might experience high shipping costs due to incorrect address validation, leading to reduced profit margins.
- Invoicing and Collections: Invalid addresses, incorrect payment terms, or missing customer data can lead to delayed payments, increased collection efforts, and strained customer relationships. In the B2B sector, inaccurate invoicing information can result in disputes, payment delays, and a growing accounts receivable backlog. Worse still, poor data quality can significantly impact bad debt recovery—if you have incorrect information, pursuing legal action to retrieve unpaid debts becomes nearly impossible. This not only affects your cash flow but also exposes your business to greater financial risk.
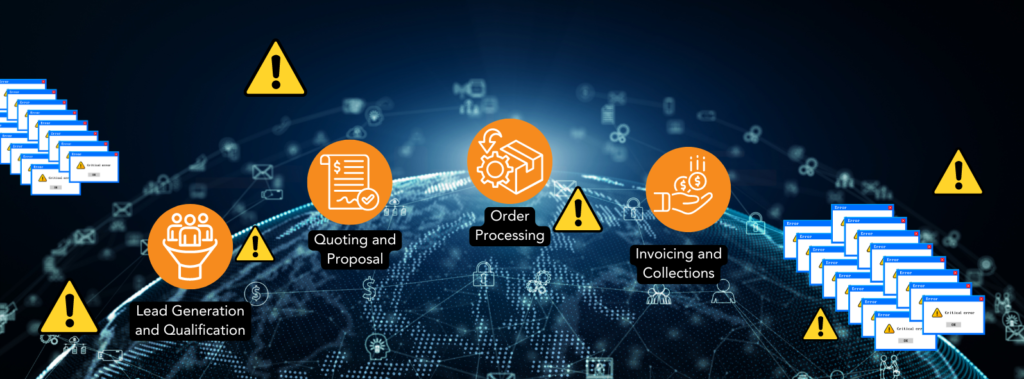
Integration Issues Amplified By Poor Data Quality
- CRM and ERP Mismatch: Inconsistent customer data between these systems can lead to order discrepancies, delayed invoicing, and inaccurate sales forecasting.
- Data Migration Errors: Moving poor-quality data from legacy systems to a new platform without proper cleansing and remediation can propagate errors and inconsistencies, compromising the integrity of your data on the new system.
- Analytics and Reporting Challenges: Inaccurate or incomplete data can lead to misleading insights, hindering data-driven decision making.
The High Cost Of Dirty Data
- Lost Revenue: Inaccurate quotes, delayed orders, and payment issues can result in lost sales and reduced revenue. A study by Gartner found that poor data quality costs organizations an average of 15% of their revenue annually.
- Increased Costs: Manual data corrections, dispute resolution, and chargebacks can drive up operational costs. For example, a manufacturing company might incur significant costs due to rework caused by incorrect product specifications resulting from poor data quality.
- Damaged Customer Relationships: Errors and delays caused by poor data quality can erode customer trust and loyalty. In the service industry, incorrect customer information can lead to poor service experiences, resulting in customer churn.
Real World Examples Of Bad Data Quality
- Equifax’s Credit Score Debacle: In 2022, Equifax, a leading credit reporting agency, faced a significant issue when millions of consumers received inaccurate credit scores due to a coding error in a legacy server. These errors, with deviations as large as 20 points, potentially affected individuals’ ability to secure loans, credit cards, and even employment.
- Public Health England’s COVID-19 Data Glitch: At the height of the COVID-19 pandemic, Public Health England (PHE) failed to report thousands of positive cases due to a technical glitch in their data recording system. This underreporting skewed infection rates and undermined public health efforts, highlighting the critical importance of accurate data in crisis management.
- Volkswagen’s Emissions Scandal: Volkswagen’s deliberate manipulation of emissions data for its diesel vehicles led to billions in fines and severely damaged the company’s brand. This scandal underscores the perils of data manipulation for short-term gains, demonstrating that poor data quality can stem from both unintended errors and intentional misconduct.
- Facebook’s Misleading Metrics and Targeted Advertising: Facebook has faced ongoing criticism for its use of misleading metrics and inaccurate user data in targeted advertising. For instance, in 2017, it was revealed that the platform had overestimated the average time users spent watching videos, causing advertisers to base decisions on flawed information.
How To Improve Data Quality For A Healthier L2R
- Data-driven culture: To maintain and enhance data quality, cultivating a workplace culture that prioritizes data integrity, shared ownership, and responsibility is crucial. This means embedding a data-driven mindset throughout the organization by implementing robust data management policies, standardizing data formats, and clearly defining data ownership roles to ensure accountability at every level.
- Data Cleansing: Identify and correct errors, inconsistencies, and duplicates in your data.
- Data Standardization: Establish consistent data formats and definitions across your organization.
- Data Validation: Implement rules and processes to ensure data accuracy and completeness.
- Data Enrichment: Add missing information to your data to improve its value.
- Data Governance: Establish clear data ownership, responsibilities, and policies.
Benefits Of Improved Data Quality:
- Faster L2R cycle times
- Increased sales and revenue
- Enhanced customer satisfaction
- Reduced operational costs
- Improved decision making
- Enhanced regulatory compliance
Case Study: Retail Giant Improves Sales Efficiency
A leading global retailer was struggling with low conversion rates and increased order processing costs. After a thorough data analysis, they discovered that inaccurate customer data, including incorrect shipping addresses and payment information, was causing significant issues. By implementing a robust data cleansing and validation process, the retailer was able to:
- Reduce order processing errors by 30%
- Improve on-time delivery rates by 15%
- Increase customer satisfaction scores by 10%
Case Study: Software Company Boosts Sales Pipeline
A software company experienced a decline in sales pipeline velocity due to inaccurate lead qualification data. By investing in data enrichment and cleansing, the company was able to:
- Improve lead quality by 25%
- Shorten sales cycle by 10%
- Increase sales conversion rates by 15%
By addressing data quality issues proactively, organizations can reap significant benefits and gain a competitive edge. Don’t let data be your business’s biggest obstacle. Let A5 help you navigate the choppy waters of L2R. Talk to one of our Lead-to-Revenue experts today.