The five pillars of AI Preparation help us to set the stage for the Machine Learning Development Lifecycle (MLDL) in which we build out our technical implementation of AI and NLP programs. Before you start to onboard your highly skilled team of data scientists, please rate your readiness on a scale of 1 being not prepared to 5 being more than prepared for each of the 5 pillars we are going to discuss.
Pillar 1: Data Readiness
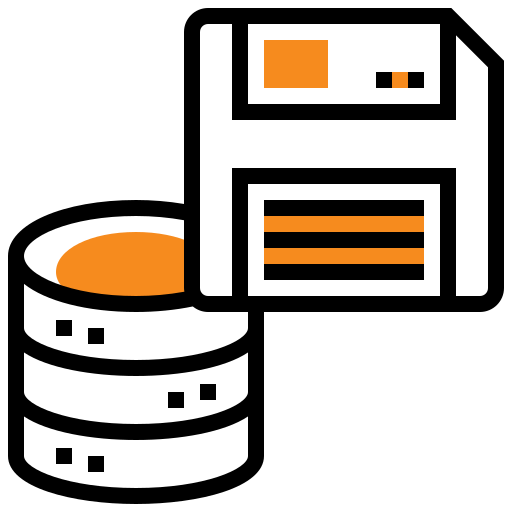
Q1: Are You Storing Data?
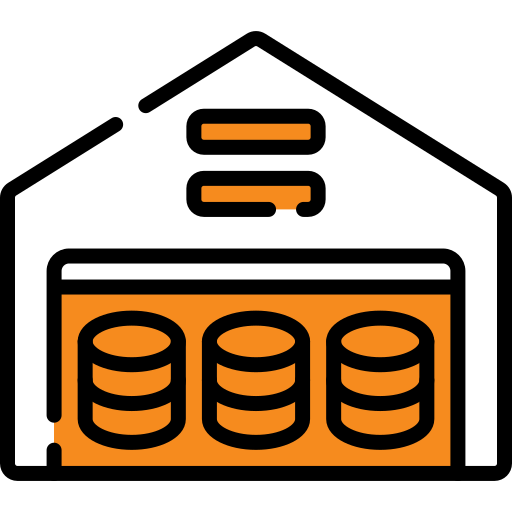
Q2: Are You Warehousing Data?
Beyond just storing data, it’s crucial to have a robust data warehousing strategy in place. This involves organizing and structuring data in a manner that makes it easily accessible for AI algorithms to extract meaningful insights. A well-designed data warehouse lays the foundation for successful AI projects.
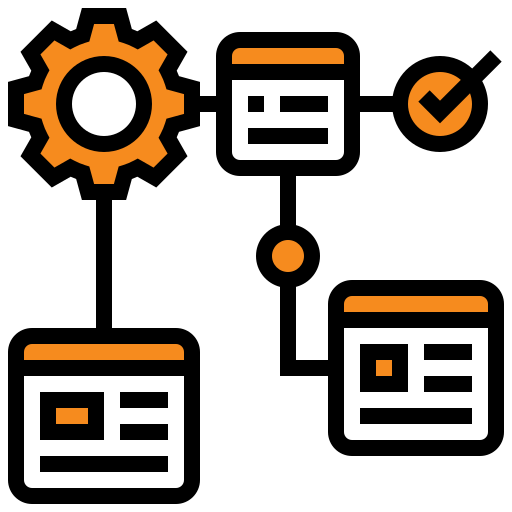
Pillar 2: Cultural readiness
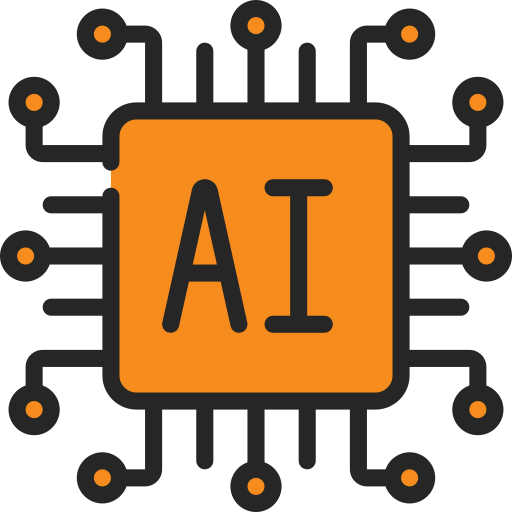
Q1: Establish AI Literacy
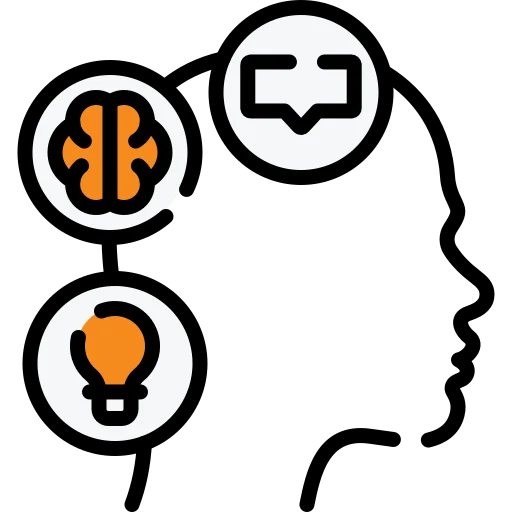
Q2: Educate Leadership
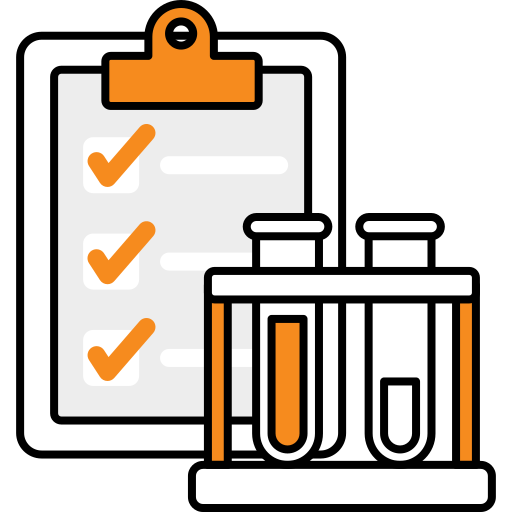
Pillar 3: Skills Readiness
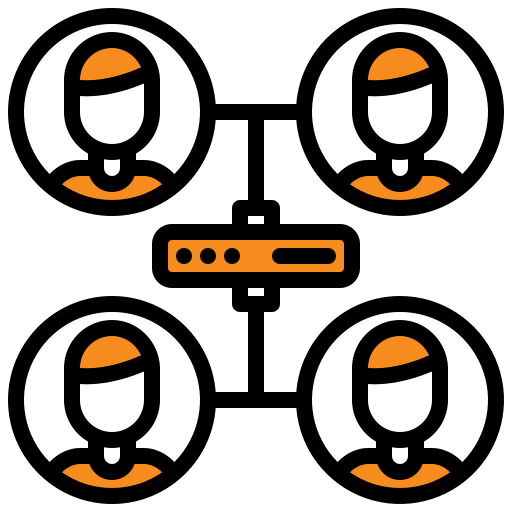
Q1: Upskill the User Base
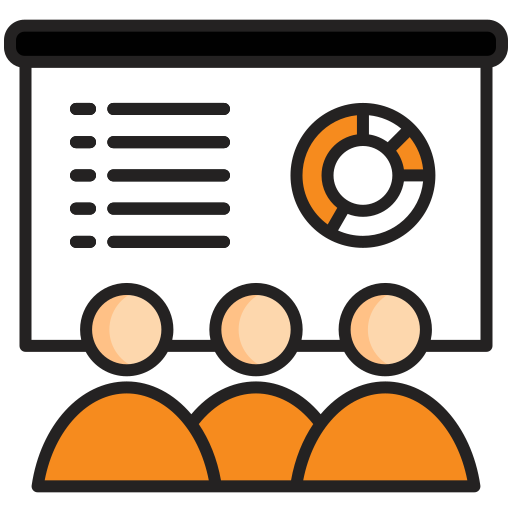
Q2: Technical Training Program
Pillar 4: Infrastructure Readiness
Infrastructure Readiness for an AI initiative refers to the state of preparedness of an organization’s technological, operational, and human resources to effectively support the implementation and deployment of artificial intelligence (AI) projects. It involves having the necessary hardware, software, data, processes, and expertise in place to leverage AI technologies efficiently and derive maximum value from them.
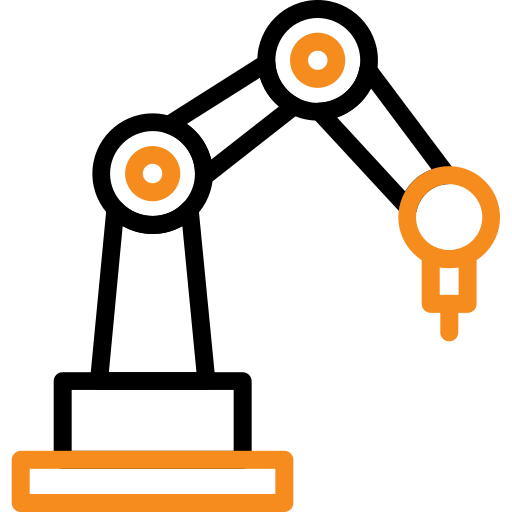
Q1: Standardize AI Infrastructure
Pillar 5: Budget Readiness
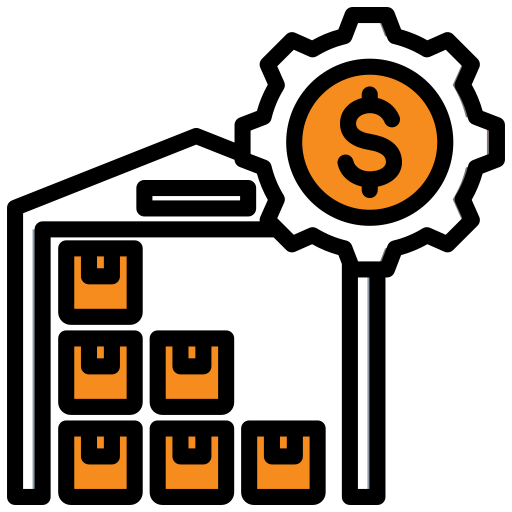
Q1: Data Warehousing Costs
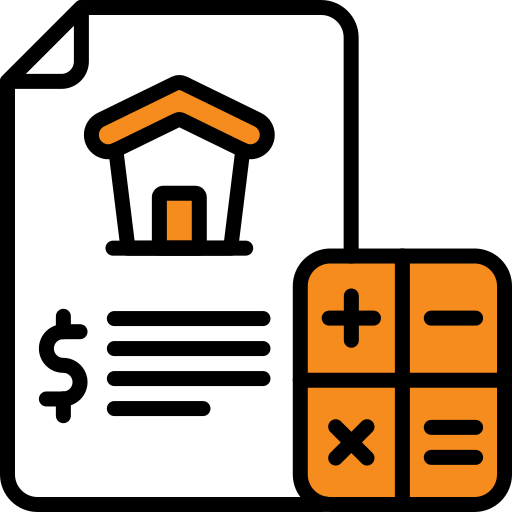
Q2: Infrastructure Costs
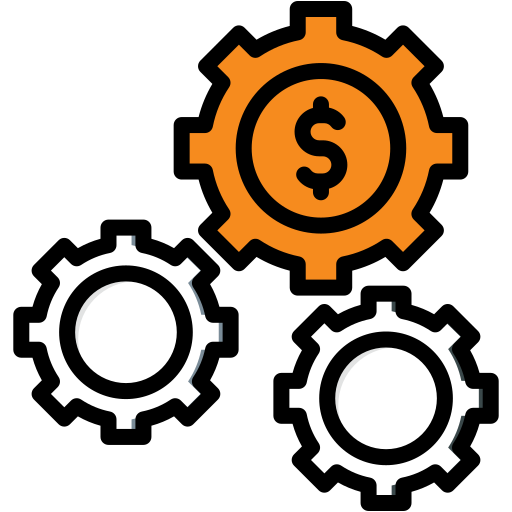
Q3: Development and Deployment Costs
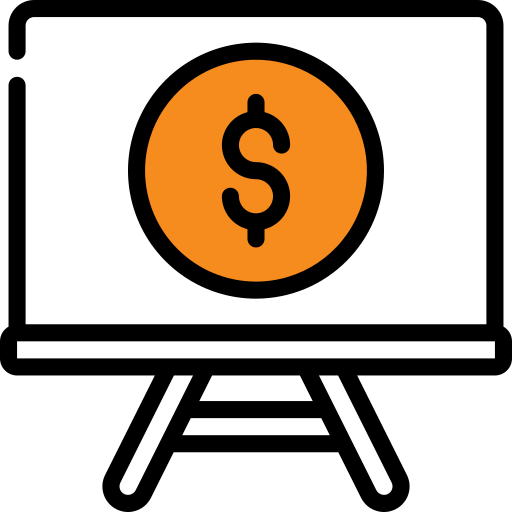
Q4: Training Costs
Training costs encompass expenses associated with upskilling employees and providing technical training programs. Organizations should budget for training initiatives to ensure their workforce is equipped to leverage AI effectively.